Today’s guest blog post is from Marcin Chudeusz of DEXT.AI. a company specializing in creating Artificial Intelligence-powered Software for Data Platforms.
Have you ever experienced the frustration of missing crucial pieces in your data puzzle? The feeling of the weight of responsibility on your shoulders when data issues suddenly arise and the entire organization looks to you to save the day? It can be overwhelming, especially when the damage has already been done. In the constantly evolving world of data management, where data warehouses, data lakes, and data lakehouses form the backbone of organizational decision-making, maintaining high-quality data is crucial. Although the challenges of managing data quality in these environments are many, the solutions, while not always straightforward, are within reach.
Data warehouses, data lakes, and lakehouses each encounter their own unique data quality challenges. These challenges range from integrating data from various sources, ensuring consistency, and managing outdated or irrelevant data, to handling the massive volume and variety of unstructured data in data lakes, which makes standardizing, cleaning, and organizing data a daunting task.
Today, I would like to introduce you to Digna, your AI-powered guardian for data quality that’s about to revolutionize the game! Get ready for a journey into the world of modern data management, where every twist and turn holds the promise of seamless insights and transformative efficiency.
Digna: A New Dawn in Data Quality Management
Picture this: you’re at the helm of a data-driven organization, where every byte of data can pivot your business strategy, fuel your growth, and steer you away from potential pitfalls. Now, imagine a tool that understands your data and respects its complexity and nuances. That’s Digna for you – your AI-powered guardian for data quality.
Goodbye to Manually Defining Technical Data Quality Rules
Gone are the days when defining technical data quality rules was a laborious, manual process. You can forget the hassle of manually setting thresholds for data quality metrics. Digna’s AI algorithm does it for you, defining acceptable ranges and adapting as your data evolves. Digna’s AI learns your data, understands it, and sets the rules for you. It’s like having a data scientist in your pocket, always working, always analyzing.

Figure 1: Learn how Digna’s AI algorithm defines acceptable ranges for data quality metrics like missing values. Here, the ideal count of missing values should be between 242 and 483, and how do you manually define technical rules for that?
Seamless Integration and Real-time Monitoring
Imagine logging into your data quality tool and being greeted with a comprehensive overview of your week’s data quality. Instant insights, anomalies flagged, and trends highlighted – all at your fingertips. Digna doesn’t just flag issues; it helps you understand them. Drill down into specific days, examine anomalies, and understand the impact on your datasets.
Whether you’re dealing with data warehouses, data lakes, or lakehouses, Digna slips in like a missing puzzle piece. It connects effortlessly to your preferred database, offering a suite of features that make data quality management a breeze. Digna’s integration with your current data infrastructure is seamless. Choose your data tables, set up data retrieval, and you’re good to go.
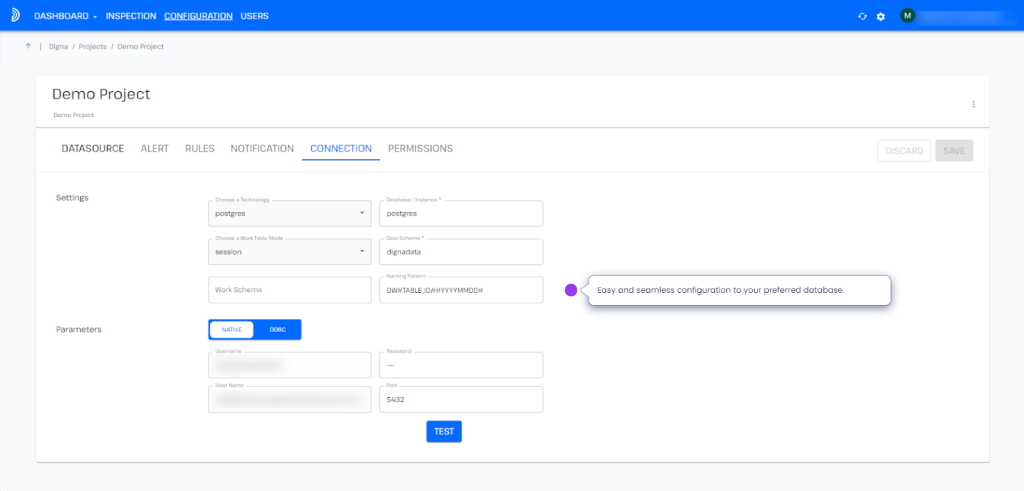
Figure 2: Connect seamlessly to your preferred database. Select specific tables from your database for detailed analysis by Digna.
Navigate Through Time and Visualize Data Discrepancies
With Digna, the journey through your data’s past is as simple as a click. Understand how your data has evolved, identify patterns, and make informed decisions with ease. Digna’s charts are not just visually appealing; they’re insightful. They show you exactly where your data deviated from expectations, helping you pinpoint issues accurately.
Read also: Navigating the Landscape – Moden Data Quality with Digna
Digna’s Holistic Observability with Minimal Setup
With Digna, every column in your data table gets attention. Switch between columns, unravel anomalies, and gain a holistic view of your data’s health. It doesn’t just monitor data values; it keeps an eye on the number of records, offering comprehensive analysis and deep insights with minimal configuration. Digna’s user-friendly interface ensures that you’re not bogged down by complex setups.

Figure 3: Observe how Digna tracks not just data values but also the number of records for comprehensive analysis. Transition seamlessly to Dataset Checks and witness Digna’s learning capabilities in recognizing patterns.
Real-time Personalized Alert Preferences
Digna’s alerts are intuitive and immediate, ensuring you’re always in the loop. These alerts are easy to understand and come in different colors to indicate the quality of the data. You can customize your alert preferences to match your needs, ensuring that you never miss important updates. With this simple yet effective system, you can quickly assess the health of your data and stay ahead of any potential issues. This way, you can avoid real-life impacts of data challenges.
Kickstart your Modern Data Quality Journey
Whether you prefer inspecting your data directly from the dashboard or integrating it into your workflow, I invite you to commence your data quality journey. It’s more than an inspection; it’s an exploration—an adventure into the heart of your data with a suite of features that considers your data privacy, security, scalability, and flexibility.
Automated Machine Learning
Digna leverages advanced machine learning algorithms to automatically identify and correct anomalies, trends, and patterns in data. This level of automation means that Digna can efficiently process large volumes of data without human intervention, erasing errors and increasing the speed of data analysis.
The system’s ability to detect subtle and complex patterns goes beyond traditional data analysis methods. It can uncover insights that would typically be missed, thus providing a more comprehensive understanding of the data.
This feature is particularly useful for organizations dealing with dynamic and evolving data sets, where new trends and patterns can emerge rapidly.
Domain Agnostic
Digna’s domain-agnostic approach means it is versatile and adaptable across various industries, such as finance, healthcare, and telcos. This versatility is essential for organizations that operate in multiple domains or those that deal with diverse data types.
The platform is designed to understand and integrate the unique characteristics and nuances of different industry data, ensuring that the analysis is relevant and accurate for each specific domain.
This adaptability is crucial for maintaining accuracy and relevance in data analysis, especially in industries with unique data structures or regulatory requirements.
Data Privacy
In today’s world, where data privacy is paramount, Digna places a strong emphasis on ensuring that data quality initiatives are compliant with the latest data protection regulations.
The platform uses state-of-the-art security measures to safeguard sensitive information, ensuring that data is handled responsibly and ethically.
Digna’s commitment to data privacy means that organizations can trust the platform to manage their data without compromising on compliance or risking data breaches.
Built to Scale
Digna is designed to be scalable, accommodating the evolving needs of businesses ranging from startups to large enterprises. This scalability ensures that as a company grows and its data infrastructure becomes more complex, Digna can continue to provide effective data quality management.
The platform’s ability to scale helps organizations maintain sustainable and reliable data practices throughout their growth, avoiding the need for frequent system changes or upgrades.
Scalability is crucial for long-term data management strategies, especially for organizations that anticipate rapid growth or significant changes in their data needs.
Real-time Radar
With Digna’s real-time monitoring capabilities, data issues are identified and addressed immediately. This prompt response prevents minor issues from escalating into major problems, thus maintaining the integrity of the decision-making process.
Real-time monitoring is particularly beneficial in fast-paced environments where data-driven decisions need to be made quickly and accurately.
This feature ensures that organizations always have the most current and accurate data at their disposal, enabling them to make informed decisions swiftly.
Choose Your Installation
Digna offers flexible deployment options, allowing organizations to choose between cloud-based or on-premises installations. This flexibility is key for organizations with specific needs or constraints related to data security and IT infrastructure.
Cloud deployment can offer benefits like reduced IT overhead, scalability, and accessibility, while on-premises installation can provide enhanced control and security for sensitive data.
This choice enables organizations to align their data quality initiatives with their broader IT and security strategies, ensuring a seamless integration into their existing systems.
Conclusion
Addressing data quality challenges in data warehouses, lakes, and lakehouses requires a multifaceted approach. It involves the integration of cutting-edge technology like AI-powered tools, robust data governance, regular audits, and a culture that values data quality.
Digna is not just a solution; it’s a revolution in data quality management. It’s an intelligent, intuitive, and indispensable tool that turns data challenges into opportunities.
I’m not just proud of what we’ve created at DEXT.AI; I’m most excited about the potential it holds for businesses worldwide. Join us on this journey, schedule a call with us, and let Digna transform your data into a reliable asset that drives growth and efficiency.
Cheers to modern data quality at scale with Digna!
This article was written by Marcin Chudeusz, CEO and Co-Founder of DEXT.AI. a company specializing in creating Artificial Intelligence-powered Software for Data Platforms. Our first product, Digna offers cutting-edge solutions through the power of AI to modern data quality issues.
Contact me to discover how Digna can revolutionize your approach to data quality and kickstart your journey to data excellence.